The Data Science Revolution in Finance – From Fraud Foiling to Self-Optimizing Portfolios
- Sam workspace
- Mar 19
- 3 min read
The banking sector is undergoing a quantum leap in operational intelligence, powered by data science architectures that process petabytes of transactional, behavioral, and regulatory data in milliseconds. Three seismic shifts are redefining finance: real-time fraud ecosystems that outthink criminals, autonomous portfolio engines that rewrite investment strategies hourly, and compliance systems that translate Basel IV regulations into executable code.
Fraud Detection Ecosystems: Adaptive Neural Nets vs. Financial Crime
Modern fraud systems employ ensemble machine learning models that combine supervised learning on historical fraud patterns with unsupervised anomaly detection. Key innovations in 2025:
Real-Time Threat Adaptation Architecture
Graph Neural Networks mapping 360-degree relationship webs between accounts, devices, and locations
Reinforcement Learning agents simulating attacker behavior to preempt novel fraud vectors
Federated Learning pools insights from 300+ banks without sharing sensitive customer data
Metric | Traditional Systems (2020) | AI-Driven Ecosystems (2025) |
False Positives | 15:1 (fraud:legitimate flag ratio) | 2.3:1 via behavioral biometrics |
Detection Speed | 8.2 seconds per transaction | 47ms latency with edge computing |
Novel Fraud Detection | 6-9 month lag | 72-hour model retraining cycles |
Case Study – Synthetic Identity Busting: A Tier 1 bank reduced account takeover fraud by 63% using:
NLP analysis of application documents vs. dark web data dumps
Generative AI creating synthetic fraud attempts to stress-test systems
Real-time device fingerprinting with 99.8% uniqueness confidence
AI Portfolio Managers: The Rise of Self-Optimizing Investment Engines
Data science has birthed portfolio systems that rebalance assets using:
Alternative Data Ingestion: Processing satellite imagery, supply chain IoT data, and social sentiment at petabyte scale
Multi-Armed Bandit Algorithms: Continuously testing strategy variations across market regimes
Explainable AI (XAI): Generating SEC-compliant rationale for each trade
Dynamic Strategy Execution in Practice:
BlackRock's Aladdin 2.1: Processes 1.2M macroeconomic indicators to adjust hedge ratios every 53 seconds
GPIF's Manager Selection AI: Improved active manager selection accuracy by 29% using style drift detection algorithms
JPMorgan's Liquidity Oracle: Predicts bond market depth with 94% accuracy using limit order book simulations
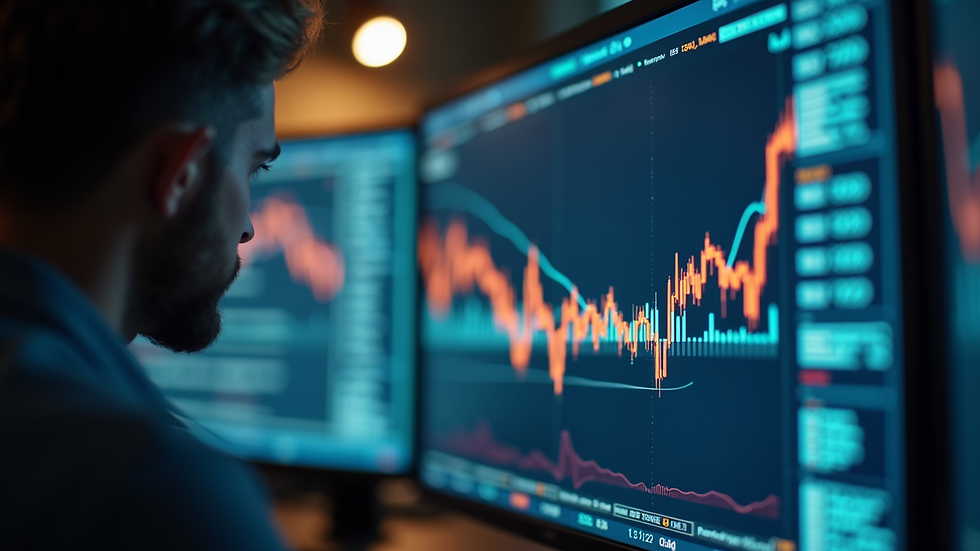
Regulatory Bots: From Basel IV Text to Automated Compliance Code
The Basel IV complexity surge (700+ risk calculation updates) is being tamed by:
Autonomous Compliance Stack
Regulatory NLP: Transformer models converting 2600-page guidelines into machine-executable rules
Smart Contract Audits: Real-time transaction scoring against capital adequacy requirements
Quantum-Enhanced Monte Carlo: Running 18M risk scenarios nightly vs. traditional 90K
Implementation Impact:
45% reduction in data quality errors through AI validation pipelines
30% faster MiFID III reporting via automated XBRL tagging
$17M annual savings per mid-sized bank in compliance staffing cost
Challenges in the Data-Driven Finance Era
Model Risk Management: 42% of AI credit models show unacceptable racial bias drift post-deployment
Data Lineage Complexity: Tracing 157-step data transformations for audit trails
Edge Computing Security: 2.3X increase in adversarial attacks on real-time fraud APIs
The Next Frontier: Banking's Quantum Leap
Leading institutions are piloting:
Homomorphic Encryption: Training fraud models on encrypted transaction streams
Neuromorphic Chips: Processing derivative pricing 900X faster than GPU clusters
Ethical AI Governors: Autonomous systems auditing model fairness using causal inference
As Citi's Chief Data Scientist recently noted:
"Our AI doesn't just detect money laundering – it predicts which regulatory changes will pass six months before legislators vote. Data science isn't supporting finance anymore; it's redefining it."
By 2027, data science-powered banking could achieve:
$412B annual reduction in global fraud losses
35% alpha generation from alternative data-driven strategies
0 manual interventions in Basel IV capital reporting
The future of finance isn't just digital – it's decisively, irreversibly algorithmic.
Comments